Machine Learning in Agriculture: A Path to Sustainability
Machine Learning
5 MIN READ
March 18, 2025
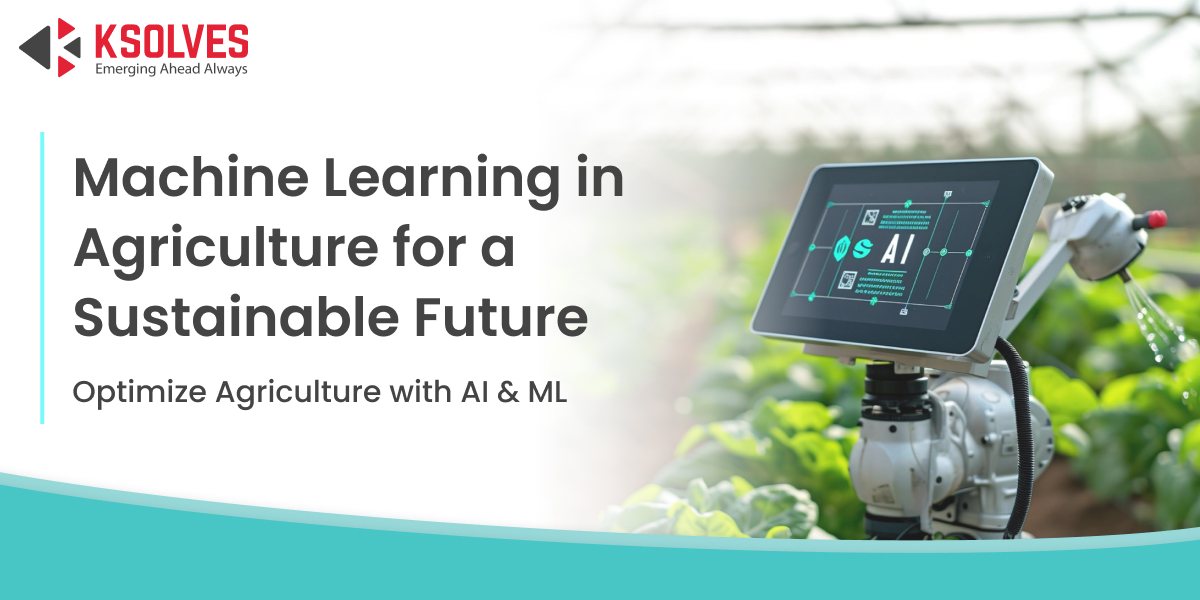
How Machine Learning is Reshaping Modern Agriculture for a Sustainable Future
Agriculture, the backbone of several economies, is experiencing extraordinary change. As the population increases and so does the need for food, conventional farming practices face extreme difficulty meeting production targets.
Machine Learning is a revolutionary technology that offers actionable insights to optimize agricultural yield. By analyzing large data sets, ML can predict crop outcomes, optimize resource allocation, and enable farmers to make informed decisions, thereby ensuring better productivity and sustainability.
This blog explores how ML reshapes agriculture, focusing on yield prediction to empower farmers. ML provides tools to mitigate risks and maximize profits, from understanding soil health to forecasting weather patterns.
If you’re considering integrating technology into your agricultural business, this guide will unveil how ML can transform your fields into hubs of innovation and efficiency.
Understanding Agricultural Yield Prediction
Yield prediction using ML refers to the process of estimating the amount of crop output a specific area of land will produce. This process involves analyzing various factors such as weather patterns, soil quality, pest activity, and irrigation practices to forecast potential yields. Accurate predictions are vital for optimizing agricultural strategies and ensuring efficient resource utilization.
Why Accurate Yield Prediction is Crucial for Farmers and Agri-businesses
1. Informed Planning
Accurate predictions enable farmers to plan critical agricultural activities, such as planting, irrigation, and harvesting. This ensures operations align with optimal growing conditions, improving overall productivity and profitability.
2. Risk Mitigation
With precise forecasts, farmers can anticipate challenges like unfavorable weather conditions, pest outbreaks, or resource shortages. Early awareness allows them to take proactive measures, minimizing potential losses.
3. Optimized Resource Allocation
Knowing expected yields helps in the efficient allocation of limited resources such as water, fertilizers, pesticides, and labor. This not only reduces waste but also lowers operational costs.
4. Improved Supply Chain Management
Yield predictions help streamline supply chains by forecasting production volumes. This ensures timely market deliveries, reducing post-harvest losses and stabilizing food availability.
5. Economic Stability for Farmers and Agribusinesses
Accurate predictions reduce the uncertainties in farming, offering financial security to farmers. Agribusinesses also benefit from stable supply forecasts, facilitating better pricing and contract planning.
6. Adaptation to Climate Change
As climate change alters agricultural patterns, yield predictions provide the insights needed to adapt practices to new conditions, ensuring sustainable farming.
7. Global Food Security
Accurate predictions support efforts to meet growing food demands by maximizing efficiency and minimizing waste. This ensures a steady food supply to a rising global population.
In essence, accurate yield prediction empowers farmers and agribusinesses with the foresight to make informed, strategic decisions, fostering resilience, sustainability, and profitability in the agricultural sector.
Key Factors Influencing Crop Yield
Several interconnected factors affect crop yield:
- Weather: Rainfall, temperature, and sunlight directly influence crop growth.
- Soil Quality: Nutrient content, pH levels, and moisture retention are critical for healthy crops.
- Pest Attacks: Infestations can significantly reduce output if not detected early.
- Irrigation: Proper water management is essential to avoid under or over-watering.
Machine Learning in Agriculture: A Revolutionary Approach
Machine Learning is transforming agriculture by introducing advanced methods for analyzing data and predicting outcomes. This revolutionary approach is reshaping farming practices and decision-making.
Benefits of Machine Learning in Agriculture
- Accurate Yield Predictions
ML algorithms analyze historical data, weather patterns, and soil conditions to predict crop yields with precision, enabling better planning and resource allocation. - Optimized Resource Usage
ML-powered systems provide real-time recommendations for water, fertilizers, and pesticides, reducing wastage and ensuring optimal resource utilization. - Early Pest and Disease Detection
With advanced image recognition and predictive analytics, ML identifies early signs of pest infestations and diseases, enabling timely intervention and minimizing crop losses. - Improved Decision-Making
ML provides actionable insights based on data trends, helping farmers make informed decisions about planting schedules, crop rotation, and irrigation. - Cost Reduction
By optimizing resources and reducing losses, ML significantly lowers operational costs, making farming more profitable for small and large-scale farmers alike. - Weather Forecasting and Adaptation
ML models use weather data to predict and prepare for climatic changes, ensuring farmers can adapt and mitigate risks associated with unpredictable weather. - Enhanced Crop Quality
ML systems monitor plant health and recommend measures to improve the quality of the produce, leading to better market value and customer satisfaction. - Efficient Supply Chain Management
By predicting yield volumes accurately, ML aids in streamlining logistics and reducing post-harvest losses, ensuring produce reaches the market on time. - Scalable Precision Farming
ML enables precision farming practices, where every field and crop is treated individually, maximizing yield while maintaining environmental sustainability. - Increased Sustainability
ML promotes environmentally friendly practices by minimizing chemical usage and encouraging the efficient use of natural resources, contributing to long-term agricultural sustainability.
Key Machine Learning Techniques for Yield Prediction
-
Regression Models: Predicting Crop Output Based on Historical Data
Regression models are used to predict continuous variables, such as crop yield per hectare, based on historical and current data.
How It Works:
- These models analyze past crop yields alongside influencing factors like rainfall, temperature, soil quality, and irrigation.
- The relationships between variables are used to predict future yields under similar conditions.
Applications:
- Estimating total harvest for resource planning.
- Identifying factors contributing most significantly to yield variations.
-
Classification Models: Identifying High-Yield Zones
Classification models categorize fields or regions into distinct groups, such as high, medium, or low-yield zones.
How It Works:
- The algorithm evaluates features such as soil type, topography, and weather patterns to classify areas based on their productivity potential.
Applications:
- Zoning fields for precision farming.
- Allocating resources efficiently to areas with higher yield potential.
-
Time Series Analysis: Forecasting Based on Seasonal Patterns
Time series models are used to analyze data trends over time and forecast future outcomes based on historical seasonal patterns.
How It Works:
- These models consider cyclic patterns in factors like temperature, rainfall, and market demand.
- They predict future yields by analyzing seasonal dependencies.
Applications:
- Planning sowing and harvesting schedules.
- Anticipating market supply based on predicted harvest volumes.
-
Image Recognition: Monitoring Plant Health via Drone and Satellite Imagery
Image recognition techniques use visual data to assess crop health and identify issues like pest infestations or nutrient deficiencies.
How It Works:
- ML algorithms process high-resolution images captured by drones or satellites.
- They identify visual patterns or anomalies, such as discoloration or irregular growth, indicating potential problems.
Applications:
- Early detection of pests and diseases.
- Monitoring crop stress due to water or nutrient deficiencies.
- Providing real-time updates for targeted interventions.
Applications of ML in Agricultural Yield Prediction
1. Weather-Based Yield Forecasting
ML models analyze historical weather data, real-time meteorological inputs, and climate patterns to predict how weather will impact crop yields.
It helps farmers plan planting and harvesting around favorable conditions. Additionally, providing early warnings about extreme weather events hence reducing potential damage.
- Soil Analysis and Nutrient Management
ML uses data from sensors and soil tests to assess nutrient content, pH levels, and moisture. It is recommended for optimal fertilizers and amendments for specific crops. The technology helps to prevent over-fertilization and enhances soil health for long-term sustainability.
- Pest and Disease Outbreak Predictions
By analyzing environmental data, crop conditions, and pest lifecycle patterns, ML predicts outbreaks before they occur. Machine Learning enables timely intervention, reducing crop loss. Additionally, lowers dependency on blanket pesticide applications, promoting eco-friendly practices.
- Precision Farming with Real-Time Recommendations
ML systems combine data from IoT sensors, drones, and weather forecasts to provide actionable insights for individual fields.
The technology suggests precise irrigation schedules, fertilizer amounts, and pest control measures. Also, it reduces resource wastage and maximizes productivity.
The Future of Agriculture with ML
- The Role of AI and ML in Sustainable Farming
ML promotes sustainable agriculture by optimizing resource usage, minimizing environmental impact, and increasing efficiency.
Examples:
- Reducing chemical use through targeted applications.
- Conserving water by precise irrigation scheduling.
- Emerging Trends: Smart Greenhouses, Autonomous Farming, and Beyond
- Smart Greenhouses:
Use ML to control environmental factors like temperature, humidity, and light automatically, ensuring optimal growth conditions.
- Autonomous Farming:
Autonomous tractors, drones, and robots use ML for tasks like planting, weeding, and harvesting, reducing labor needs and increasing accuracy.
- Beyond:
ML-powered tools for real-time crop monitoring and predictive market analytics will further revolutionize farming.
- How ML is Shaping the Future of Global Food Security
As global food demand increases, ML helps meet this challenge by improving yield predictions, reducing waste, and enhancing productivity.
Impact:
- Provides small-scale farmers with affordable tools to compete in global markets.
- Ensures equitable food distribution by streamlining supply chains.
Conclusion
Transform your agricultural operations with cutting-edge ML solutions designed to maximize yield, optimize resources, and enhance sustainability. Whether you’re a farmer or part of an agribusiness, Ksolves is here to help you embrace technology and drive growth.
Start small, scale smart, and think big! Partner with Ksolves for machine learning consulting services and to adopt ML-powered tools tailored to your needs and step into a more productive, profitable, and sustainable agricultural future.
Contact us today to revolutionize your farming practices with ML!
AUTHOR
Machine Learning
Mayank Shukla, a seasoned Technical Project Manager at Ksolves with 8+ years of experience, specializes in AI/ML and Generative AI technologies. With a robust foundation in software development, he leads innovative projects that redefine technology solutions, blending expertise in AI to create scalable, user-focused products.
Share with